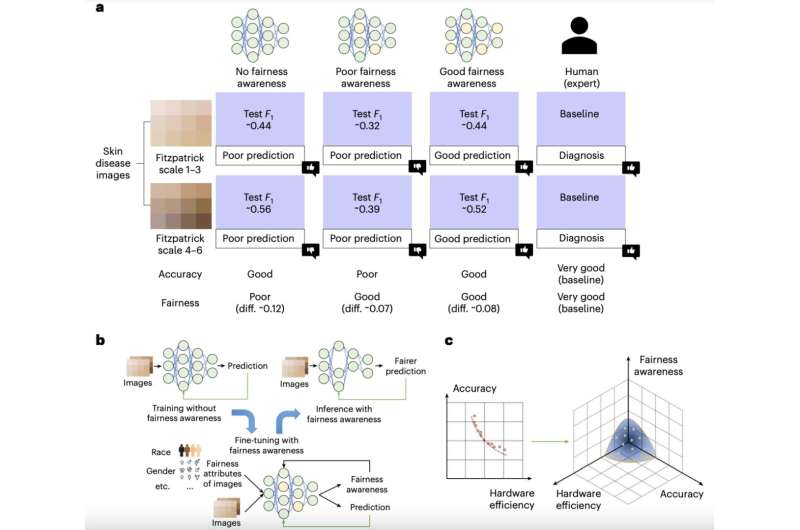
Over the previous couple of many years, pc scientists have developed a variety of deep neural networks (DNNs) designed to sort out varied real-world duties. Whereas a few of these fashions have proved to be extremely efficient, some research discovered that they are often unfair, that means that their efficiency could fluctuate based mostly on the information they have been skilled on and even the {hardware} platforms they have been deployed on.
As an illustration, some research confirmed that commercially obtainable deep studying–based mostly instruments for facial recognition have been considerably higher at recognizing the options of fair-skinned people in comparison with dark-skinned people. These noticed variations within the efficiency of AI, in nice half because of disparities within the coaching information obtainable, have impressed efforts aimed toward enhancing the equity of current fashions.
Researchers at College of Notre Dame not too long ago got down to examine how {hardware} methods can contribute to the equity of AI. Their paper, printed in Nature Electronics, identifies methods through which rising {hardware} designs, akin to computing-in-memory (CiM) units, can have an effect on the equity of DNNs.
“Our paper originated from an pressing want to deal with equity in AI, particularly in high-stakes areas like well being care, the place biases can result in important hurt,” Yiyu Shi, co-author of the paper, informed Tech Xplore.
“Whereas a lot analysis has targeted on the equity of algorithms, the function of {hardware} in influencing equity has been largely ignored. As AI fashions more and more deploy on resource-constrained units, akin to cellular and edge units, we realized that the underlying {hardware} may probably exacerbate or mitigate biases.”
After reviewing previous literature exploring discrepancies in AI efficiency, Shi and his colleagues realized that the contribution of {hardware} design to AI equity had not been investigated but. The important thing goal of their latest examine was to fill this hole, particularly analyzing how new CiM {hardware} designs affected the equity of DNNs.
“Our intention was to systematically discover these results, notably via the lens of rising CiM architectures, and to suggest options that might assist guarantee truthful AI deployments throughout numerous {hardware} platforms,” Shi defined. “We investigated the connection between {hardware} and equity by conducting a sequence of experiments utilizing totally different {hardware} setups, notably specializing in CiM architectures.”
As a part of this latest examine, Shi and his colleagues carried out two predominant kinds of experiments. The primary sort was aimed toward exploring the impression of hardware-aware neural structure designs various in dimension and construction, on the equity of the outcomes attained.
“Our experiments led us to a number of conclusions that weren’t restricted to machine choice,” Shi mentioned. “For instance, we discovered that bigger, extra complicated neural networks, which usually require extra {hardware} assets, are inclined to exhibit better equity. Nonetheless, these higher fashions have been additionally harder to deploy on units with restricted assets.”
Constructing on what they noticed of their experiments, the researchers proposed potential methods that might assist to extend the equity of AI with out posing important computational challenges. One attainable answer might be to compress bigger fashions, thus retaining their efficiency whereas limiting their computational load.
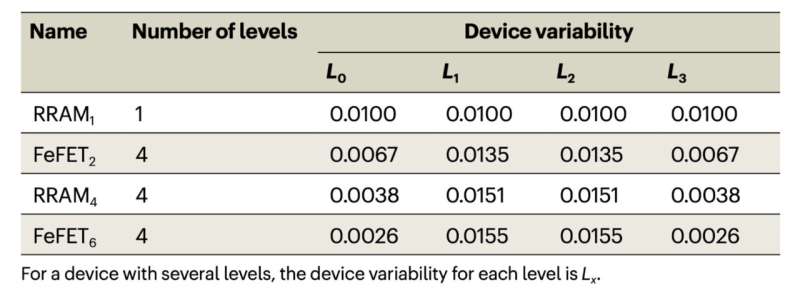
“The second sort of experiments we carried out targeted on sure non-idealities, akin to machine variability and stuck-at-fault points coming along with CiM architectures,” Shi mentioned. “We used these {hardware} platforms to run varied neural networks, analyzing how modifications in {hardware}—akin to variations in reminiscence capability or processing energy—affected the mannequin‘s equity.
“The outcomes confirmed that varied trade-offs have been exhibited below totally different setups of machine variations and that current strategies used to enhance the robustness below machine variations additionally contributed to those trade-offs.”
To beat the challenges unveiled of their second set of experiments, Shi and his colleagues recommend using noise-aware coaching methods. These methods entail the introduction of managed noise whereas coaching AI fashions, as a method of enhancing each their robustness and equity with out considerably growing their computational calls for.
“Our analysis highlights that the equity of neural networks isn’t just a perform of the information or algorithms however can be considerably influenced by the {hardware} on which they’re deployed,” Shi mentioned. “One of many key findings is that bigger, extra resource-intensive fashions usually carry out higher by way of equity, however this comes at the price of requiring extra superior {hardware}.”
By way of their experiments, the researchers additionally found that hardware-induced non-idealities, akin to machine variability, can result in trade-offs between the accuracy and equity of AI fashions. Their findings spotlight the necessity to fastidiously contemplate each the design of AI mannequin buildings and the {hardware} platforms they are going to be deployed on, to succeed in an excellent stability between accuracy and equity.
“Virtually, our work means that when creating AI, notably instruments for delicate purposes (e.g., medical diagnostics), designers want to contemplate not solely the software program algorithms but additionally the {hardware} platforms,” Shi mentioned.
The latest work by this analysis staff may contribute to future efforts aimed toward growing the equity of AI, encouraging builders to concentrate on each {hardware} and software program elements. This might in flip facilitate the event of AI methods which might be each correct and equitable, yielding equally good outcomes when analyzing the information of customers with totally different bodily and ethnic traits.
“Shifting ahead, our analysis will proceed to delve into the intersection of {hardware} design and AI equity,” Shi mentioned. “We plan to develop superior cross-layer co-design frameworks that optimize neural community architectures for equity whereas contemplating {hardware} constraints. This method will contain exploring new kinds of {hardware} platforms that inherently help equity alongside effectivity.”
As a part of their subsequent research, the researchers additionally plan to plan adaptive coaching methods that might tackle the variability and limitations of various {hardware} methods. These methods may be certain that AI fashions stay truthful no matter the units they’re operating on and the conditions through which they’re deployed.
“One other avenue of curiosity for us is to analyze how particular {hardware} configurations is likely to be tuned to boost equity, probably resulting in new courses of units designed with equity as a major goal,” Shi added. “These efforts are essential as AI methods grow to be extra ubiquitous, and the necessity for truthful, unbiased decision-making turns into ever extra essential.”
Extra info:
Yuanbo Guo et al, {Hardware} design and the equity of a neural community, Nature Electronics (2024). DOI: 10.1038/s41928-024-01213-0
© 2024 Science X Community
Quotation:
How {hardware} contributes to the equity of synthetic neural networks (2024, August 24)
retrieved 24 August 2024
from https://techxplore.com/information/2024-08-hardware-contributes-fairness-artificial-neural.html
This doc is topic to copyright. Aside from any truthful dealing for the aim of personal examine or analysis, no
half could also be reproduced with out the written permission. The content material is offered for info functions solely.